AI-based model makes predicting fusion profiles faster
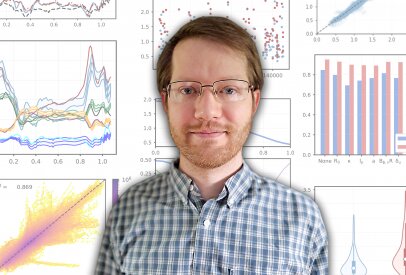
PPPL physicist Dan Boyer. (Photo: Amber Boyer/Kiran Sudarsanan)
Researchers at the Department of Energy’s Princeton Plasma Physics Laboratory are using machine learning to predict electron density and pressure profile shapes on the National Spherical Torus Experiment-Upgrade (NSTX-U), the flagship fusion facility at PPPL that is currently under repair.
The hope is that such predictions, generated by artificial neural networks, could improve the ability of NSTX-U researchers to optimize the components of experiments that heat and shape the fusion plasma.
“This is a step toward what we should do to optimize the actuators,” said PPPL physicist Dan Boyer, author of the paper, “Prediction of electron density and pressure profile shapes on NSTX-U using neural networks,” published by Nuclear Fusion, a journal of the International Atomic Energy Agency. “Machine learning can turn historical data into a simple model that we can evaluate quickly enough to make decisions in the control room or even in real time during an experiment.”